最終更新日:2025/03/08
(Featured AI in healthcare) Is it useful for glaucoma treatment? Predict the field of view with RNN! (Publication)
[論文] K. Park, J. Kim, J. Lee, “Visual Field Prediction using Recurrent Neural Network”. Scientific Reports, 9, 8385 (2019). [DOI: https://doi.org/10.1038/s41598-019-44852-6]
3つの要点
緑内障治療の意思決定に役立ちうる視野予測に取り組んだ。
シーケンスデータが入力データである。
概説
最近、AIの能力は大きく向上した。
過去数年間で深層学習アルゴリズムの一つであるリカレントニューラルネットワーク(RNN)は、シーケンスデータに対する系列ラベリングと予測タスクにおいて目立った能力を示した。
RNNを用いて信頼できる視野予測アルゴリズム(図1)を構築し、その性能を従来の点ごとの線形回帰(OLR )と比較して評価した。
合計1408個の眼を学習データ(表1)として用い、計281個の眼からなる別のデータセットをテストデータ(表2)として用いた。
構築したRNNに5つの連続した視野の検査を入力として与え、6番目の視野検査をRNNの出力と比較した。
テストデータの6番目の視野を予測することでRNNの性能をOLRの性能と比較した。
全体的なRNNの予測性能はOLRよりも有意に良好であった(図2、表3、図3)。
緑内障損傷に脆弱であることが知られているほとんどの領域において、RNNの点ごとの予測誤差はOLRのものよりも有意に小さかった。
RNNはまた、視野検査における悪化についてよりロバストで信頼性があった。
したがって臨床現場において、RNNモデルは緑内障の更なる治療のための意思決定を助けることができる。
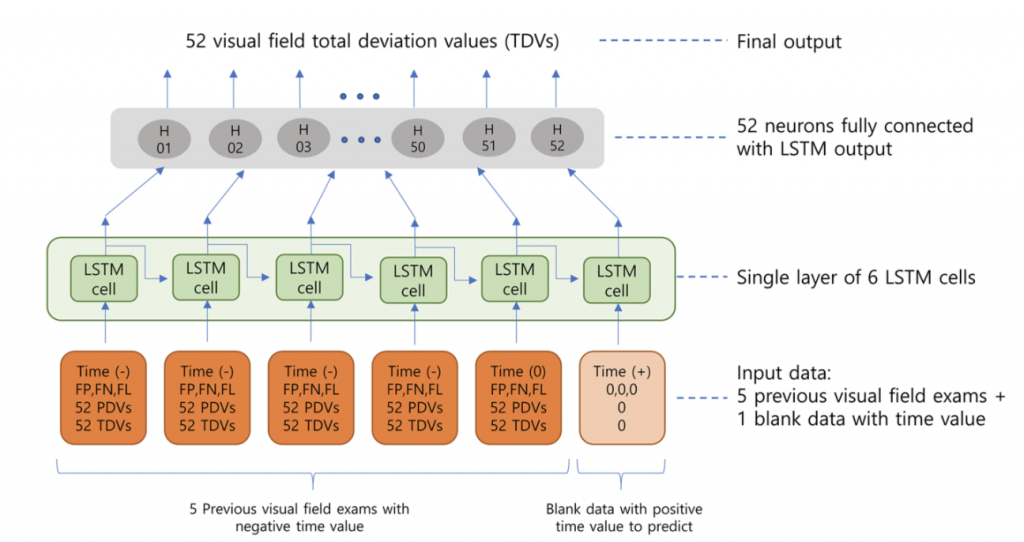
入力データは3つのカテゴリー(日にちごとの相対的時間変位、信頼性データ、視野データ)で構成されている。
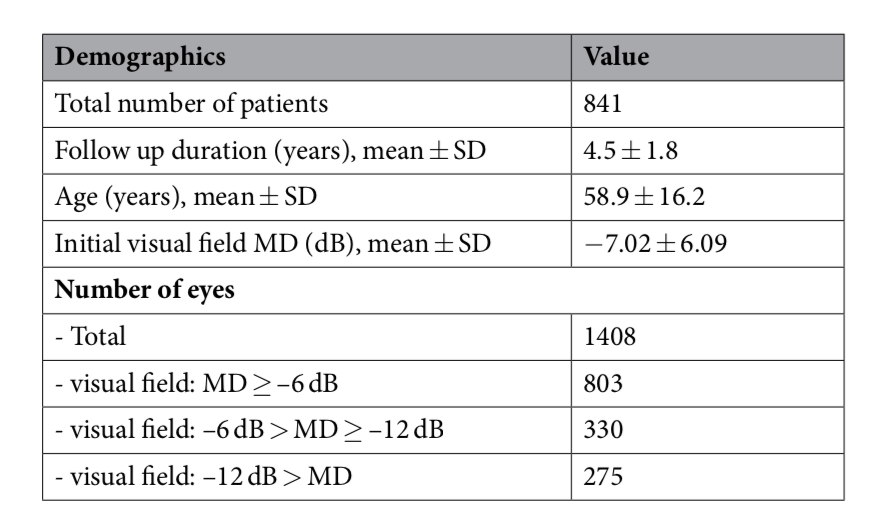
表2 テストデータの内訳。
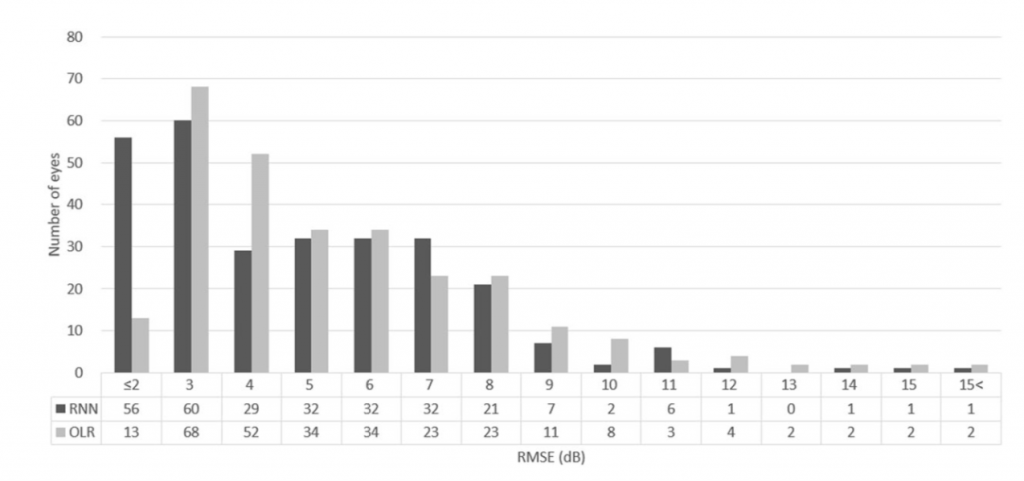
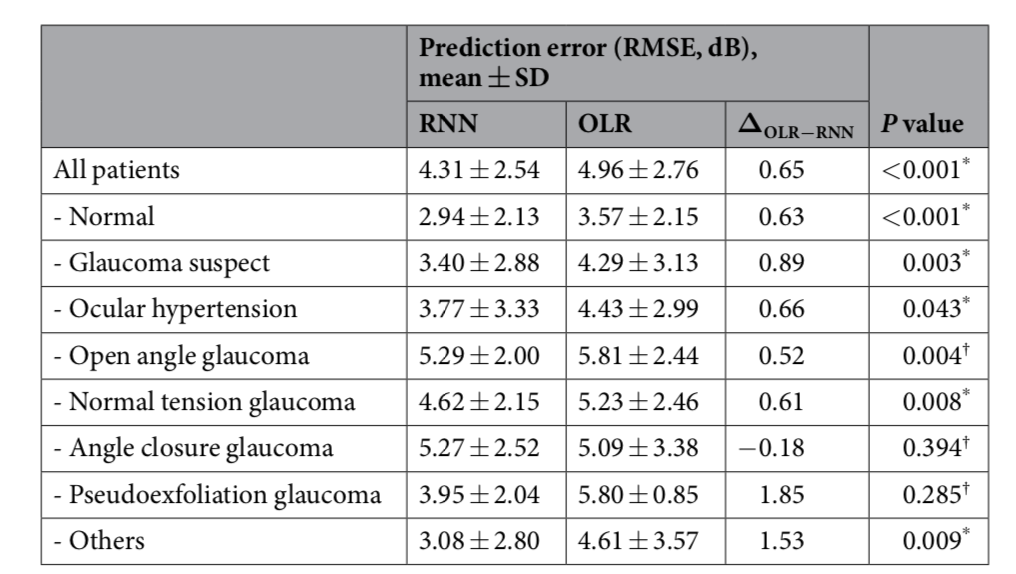
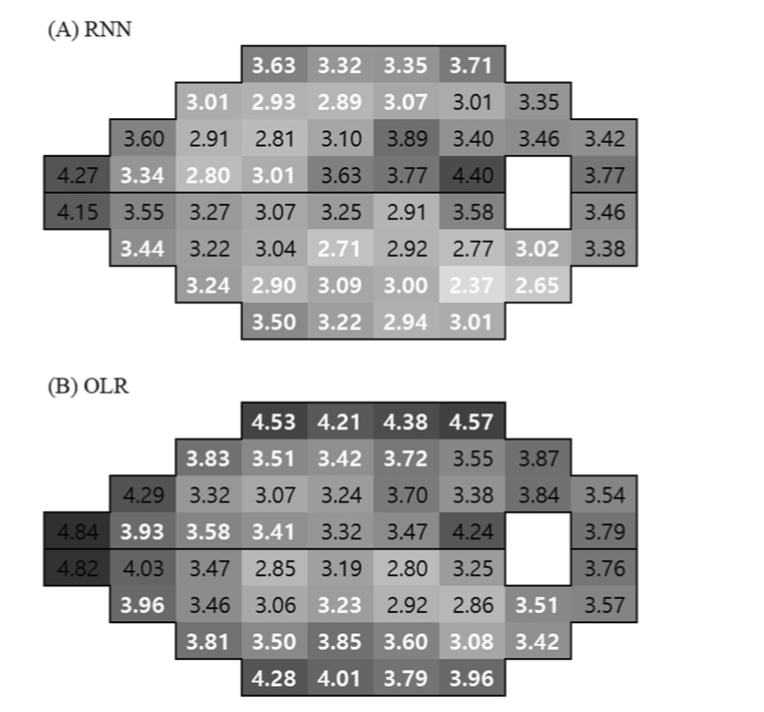
著者
Keunheung Park (Department of Ophthalmology, Pusan National University College of Medicine, Busan, Korea / Biomedical Research Institute, Pusan National University Hospital, Busan, Korea)
Jiwoong Lee (Department of Ophthalmology, Pusan National University College of Medicine, Busan, Korea / Biomedical Research Institute, Pusan National University Hospital, Busan, Korea)
Jinmi Kim (Department of Biostatistics, Clinical Trial Center, Biomedical Research Institute, Pusan National University Hospital, Busan, Korea)
出版情報
Received: 15 October 2018 / Accepted: 24 May 2019 / Published: 10 June 2019
Open Access This article is licensed under a Creative Commons Attribution 4.0 International License, which permits use, sharing, adaptation, distribution and reproduction in any medium or format, as long as you give appropriate credit to the original author(s) and the source, provide a link to the Creative Commons license, and indicate if changes were made. The images or other third party material in this article are included in the article’s Creative Commons license, unless indicated otherwise in a credit line to the material. If material is not included in the article’s Creative Commons license and your intended use is not permitted by statutory regulation or exceeds the permitted use, you will need to obtain permission directly from the copyright holder. To view a copy of this license, visit http://creativecommons.org/licenses/by/4.0/.
■サポートのお願い
AIDBを便利だと思っていただけた方に、任意の金額でサポートしていただけますと幸いです。