最終更新日:2020/03/10
[論文] Kaisar Kushibar, Sergi Valverde, Sandra González-Villà, Jose Bernal, Mariano Cabezas, Arnau Oliver and Xavier Lladó, “Supervised Domain Adaptation for Automatic Sub-cortical Brain Structure Segmentation with Minimal User Interaction”. Scientific Reports, 9, 6742 (2019). [DOI: 10.1038/s41598-019-43299-z]
3つの要点
✔️皮質化脳構造のセグメント化に畳み込みニューラルネットワーク(CNN)が応用できるとされているが、臨床現場における再現性に問題があった。
✔️転移学習戦略を採用することで、少ない画像数でも素早く学習できる手法をこの論文では提唱している。
✔️公的に利用可能なデータセットを用いたわずかな学習で、既存のアプローチより十分優れたパフォーマンスが得られることが示された。
概説
近年、磁気共鳴画像(Magnetic Resonance Imaging;MRI)から皮質下脳構造をセグメント化するために、いくつかの畳み込みニューラルネットワーク(Convolutional Neural Network;CNN)が提案されている。
これらの方法は正確なセグメンテーションを提供するが、異なる画像領域からのMRIボリュームのセグメンテーションに関する再現性の問題、例えばプロトコル、スキャナー、および強度情報の違いがある。
従って、異なる画像化領域でも同様に機能するようネットワークに最初から学習させ直さなければならず、このことは、臨床現場における適用可能性を制限してしまっている。
本論文では、領域シフト問題を解決するため、転移学習戦略を採用した。事前学習ネットワークによって得られた知識を活用することによって学習用画像の数を減らし、CNNの学習可能なパラメータの数を減らすことによって学習速度を向上させた。
公的に利用可能な2つのデータセット──MICCAI 2012とIBSR──で我々の手法をテストし、一般的に使用されているアプローチ(FIRSTとする)と比較した。我々の手法は、すべてを学習したCNNによって得られたものと同様の結果を示し、そしてターゲット領域からの使用画像数を非常に少なくした。
さらに、MICCAI 2012の1つの画像とIBSRデータセットの3つの画像のみを用いてネットワークを訓練することは、それぞれ(p < 0.001)と(p < 0.05)でFIRSTを有意に凌駕するのに十分であった。
結果は、オリジナル画像を用いた転移学習、ゼロからの学習、標準化された画像を用いた転移学習、および混合セット学習の7回の反復について示されている。緑の直線はFIRSTの結果、赤の直線は全画像を用いて完全に学習した場合、紫の直線は標準化された画像を転送学習なしでテストした結果に対応している。 すべての場合の各反復における訓練量は同じである。
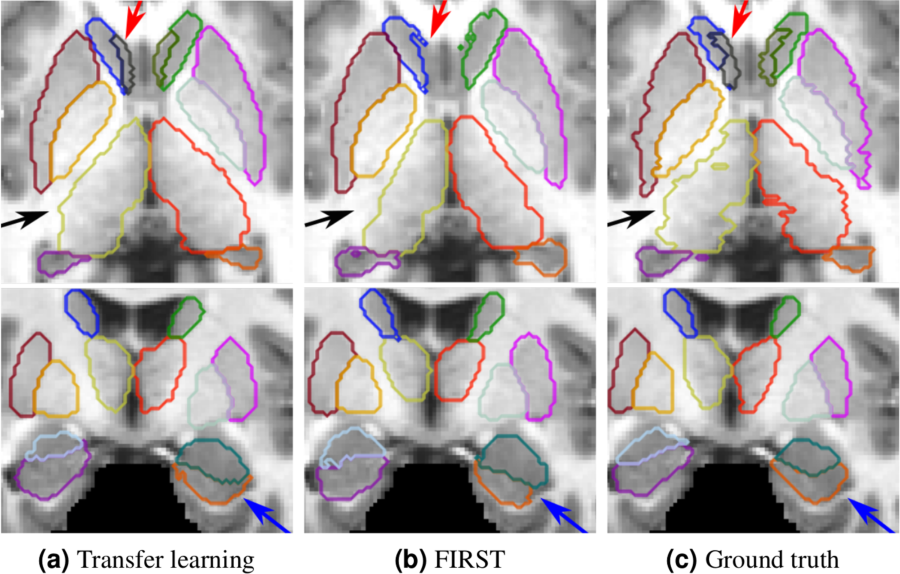
(a)1つの画像を使った転移学習
(b)FIRSTによるセグメンテーション結果
(c)Ground truth(大量の画像を用いた、根拠のある学習結果)
軸上に示された最上列と冠状面に示された最下列の結果。
矢印は以下の構造を示す:赤→側坐、黒→視床、青→海馬
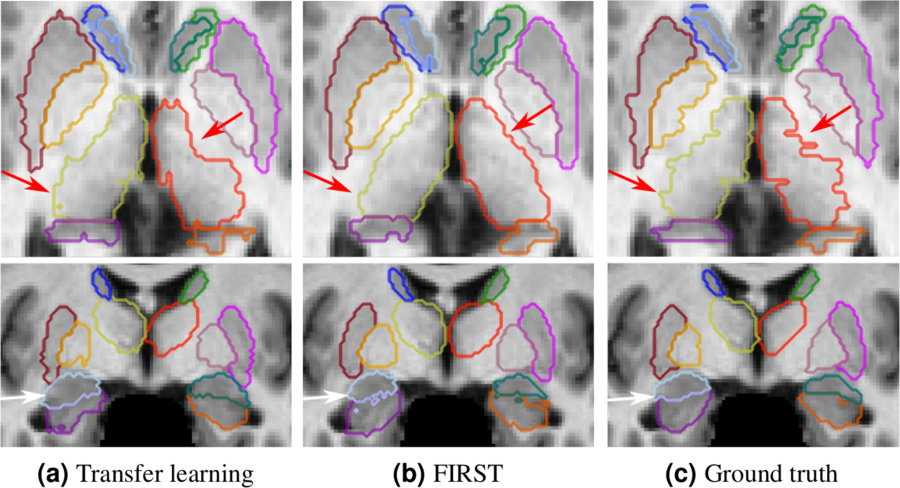
(a)3つの異なる強度分布グループからの3つの画像を用いた転移学習
(b)FIRSTによるセグメンテーション結果
(c)Ground truth(大量の画像を用いた、根拠のある学習結果)
最上列の結果は軸方向図に示され、最下列の結果は冠状図に示される。
矢印は以下を示す:赤→左右の視床、白→扁桃体および海馬の構造
著者
Kaisar Kushibar (Institute of Computer Vision and Robotics, University of Girona. Ed. P-IV, Campus Montilivi, University of Girona, 17003, Girona, Spain)
Sergi Valverde (Institute of Computer Vision and Robotics, University of Girona. Ed. P-IV, Campus Montilivi, University of Girona, 17003, Girona, Spain)
Sandra González-Villà (Institute of Computer Vision and Robotics, University of Girona. Ed. P-IV, Campus Montilivi, University of Girona, 17003, Girona, Spain)
Jose Bernal (Institute of Computer Vision and Robotics, University of Girona. Ed. P-IV, Campus Montilivi, University of Girona, 17003, Girona, Spain)
Mariano Cabezas (Institute of Computer Vision and Robotics, University of Girona. Ed. P-IV, Campus Montilivi, University of Girona, 17003, Girona, Spain)
Arnau Oliver (Institute of Computer Vision and Robotics, University of Girona. Ed. P-IV, Campus Montilivi, University of Girona, 17003, Girona, Spain)
Xavier Lladó (Institute of Computer Vision and Robotics, University of Girona. Ed. P-IV, Campus Montilivi, University of Girona, 17003, Girona, Spain)
出版情報
Received: 9 October 2018 / Accepted: 15 April 2019 / Published: 1 May 2019
Open Access This article is licensed under a Creative Commons Attribution 4.0 International License, which permits use, sharing, adaptation, distribution and reproduction in any medium or format, as long as you give appropriate credit to the original author(s) and the source, provide a link to the Creative Commons license, and indicate if changes were made. The images or other third party material in this article are included in the article’s Creative Commons license, unless indicated otherwise in a credit line to the material. If material is not included in the article’s Creative Commons license and your intended use is not permitted by statutory regulation or exceeds the permitted use, you will need to obtain permission directly from the copyright holder. To view a copy of this license, visit http://creativecommons.org/licenses/by/4.0/.
■サポートのお願い
AIDBを便利だと思っていただけた方に、任意の金額でサポートしていただけますと幸いです。