最終更新日:2025/03/08
(Featured AI and healthcare) Deep Learning is powerful tool to support cancer treatment ! Predict the survival probability of patients with metastatic cancer (Publication)
[論文] I. Banerjee, M. F. Gensheimer, D. J. Wood, S. Henry, S. Aggarwal, D. T. Chang, and D. L. Rubin, “Probabilistic Prognostic Estimates of Survival in Metastatic Cancer Patients (PPES-Met) Utilizing Free-Text Clinical Narratives”. Scientific Reports, 8, 10037 (2018). [DOI: 10.1038/s41598-018-27946-5]
3つの要点
深層学習を応用した、転移性癌患者の生存確率を推定するモデル(PPES-Met)について紹介する。
予測の根拠について医師の理解を促すツールも開発された。
PPES-Metモデルは正確でわかりやすく、医師にも患者個人にも有益なものとして使われることが期待される。
概説
一時的訪問順序が維持されている間、電子カルテのフリーテキストの臨床記録を分析することによって、患者の短期平均寿命(3ヶ月以上)を推定するための深層学習モデル──転移性癌患者における生存の確率的予後推定(PPES-Met)を提案する。
単一のフレームワークで、著者らは教師なしで異種の臨床メモから関連情報を抽出するテキスト処理法を生み出すために意味データマッピングと神経埋め込み技術を統合し、患者の訪問の時間依存性をモデル化するために回帰ニューラルネットワークを設計した。
モデルは大きなデータセット(10,293人の患者)で訓練され、別々のデータセット(1818人の患者)で検証された。
この方法では0.89のROC曲線下面積(AUC)を達成した。
説明能力を提供するために、我々はモデルの予測の根拠についての医師の理解を向上させることができる相互作用のグラフィカルツールを開発した。
PPES-Metモデルの高い正確性および説明可能性は、医師に有益な支援を提供し、転移性癌治療を個々人に合ったものにするための意思決定支援ツールとして我々のモデルが使われることを可能にするだろう。
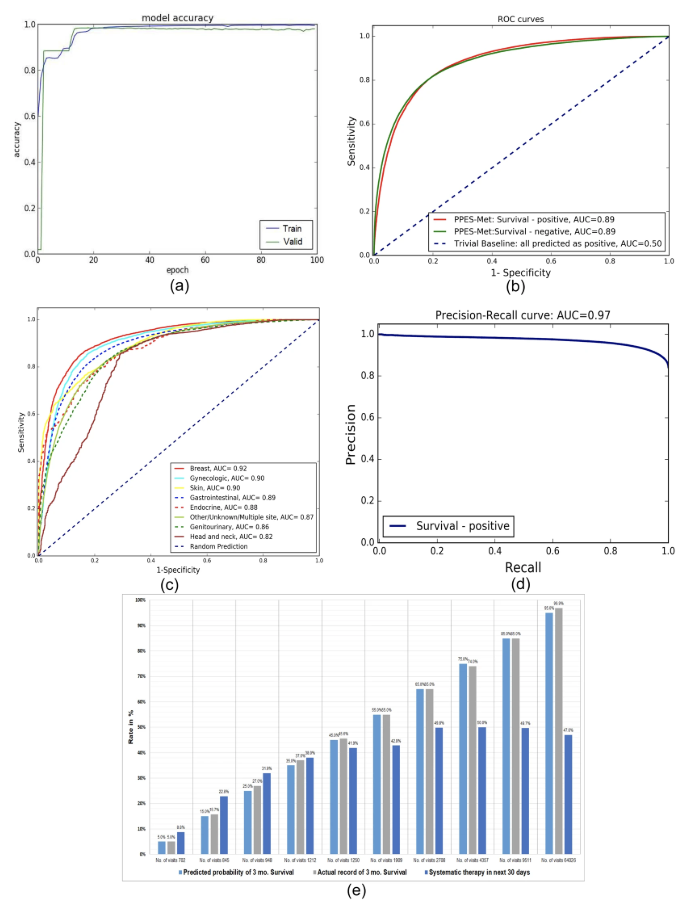
全体的な定量的パフォーマンス。
(a)エポックに基づく性能精度 (b)パディング値なしの試験セット上のROC曲線 (c)試験部位上のROC曲線を一次部位に基づいて分離 (d)パディング値を用いないテストセット上の再現精度曲線 (e)今後30日間の系統的治療情報と比較した予測確率と実際の生存率の間の相関は、高い期待生存率をもつ患者の場合は全身療法利用率のプラトーが約50%で安定している一方で、生存率が限られている場合は、多くの患者が積極的な治療を受けていることを示している。
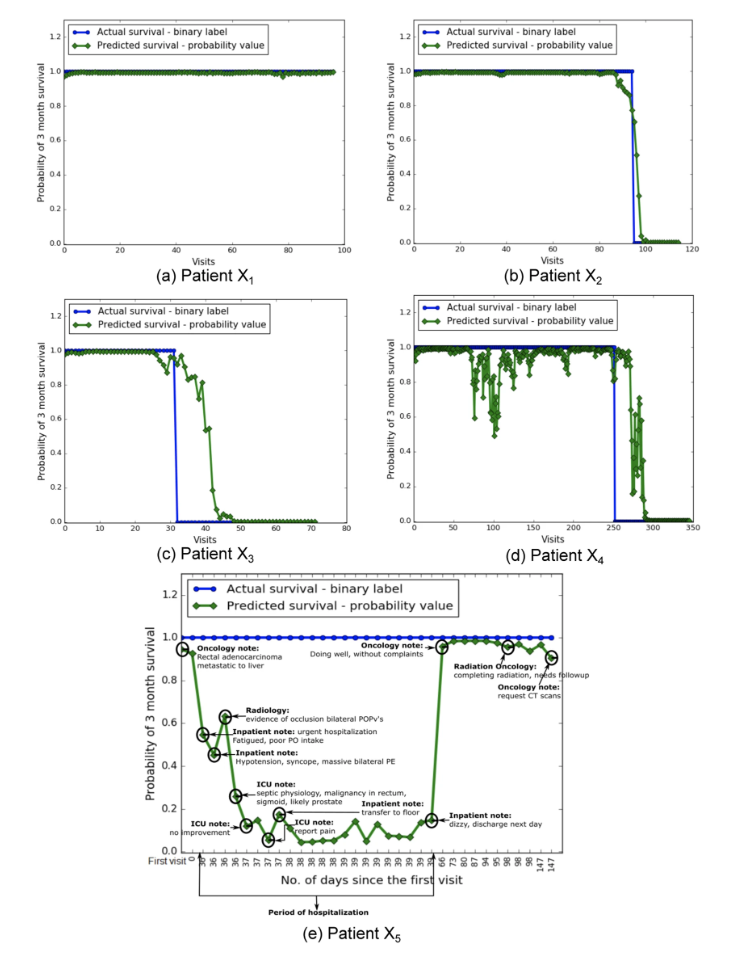
患者レベルの予後推定値 ── x軸は最初の来診から始めて昇順で来院指標を示し、y軸は生存の確率を示す。青い線はグランドトゥルース(正確さや整合性をチェックするためのバイナリーデータ)を表す。生存率が陽性なら1、陰性なら0とする。緑の線は予測生存確率スコアを表している。
(c)予測配列は、いくつかの例外を除いて実際の生存に従う (d)予測配列は、大きな変動を伴って実際の生存に従う (e)患者の明瞭な縦断生存曲線。
著者
Imon Banerjee (Department of Biomedical Data Science, Stanford University)
Michael Francis Gensheimer (Department of Radiation Oncology, Stanford University)
Douglas J. Wood (Department of Biomedical Data Science, Stanford University)
Solomon Henry (Department of Biomedical Data Science, Stanford University)
Sonya Aggarwal (Department of Radiation Oncology, Stanford University)
Daniel T. Chang (Department of Radiation Oncology, Stanford University)
Daniel L. Rubin (Department of Biomedical Data Science, Stanford University)
出版情報
Received: 1 December 2017 / Accepted: 12 June 2018 / Published: 3 July 2018
Open Access This article is licensed under a Creative Commons Attribution 4.0 International License, which permits use, sharing, adaptation, distribution and reproduction in any medium or format, as long as you give appropriate credit to the original author(s) and the source, provide a link to the Creative Commons license, and indicate if changes were made. The images or other third party material in this article are included in the article’s Creative Commons license, unless indicated otherwise in a credit line to the material. If material is not included in the article’s Creative Commons license and your intended use is not permitted by statutory regulation or exceeds the permitted use, you will need to obtain permission directly from the copyright holder. To view a copy of this license, visit http://creativecommons.org/licenses/by/4.0/.
■サポートのお願い
AIDBを便利だと思っていただけた方に、任意の金額でサポートしていただけますと幸いです。