最終更新日:2025/02/23
(Featured AI and healthcare) Can we distinguish diseases using the gut microbiome? Establishment and evaluation of machine learning-based prediction model (Publication)
[論文] S. Bang, D. Yoo, S.J. Kim, S. Jhang, S. Cho, H. Kim , “Establishment and evaluation of prediction model for multiple disease classification based on gut microbial data”. Scientific Reports 9, 10189 (2019). [DOI: 10.1038/s41598-019-46249-x]
3つの要点
✔️本研究では、機械学習を用いて、脳卒中や後天性免疫不全症候群などといった6つの疾患を腸の微生物から分類するモデルを提案している。
✔️特徴選択法に基づいて分類したところ、属レベルで分類したときに分類パフォーマンスは最も高くなることがわかった。
✔️本研究において属レベルで選択された特徴は、複合疾患の分類のためのマーカーとして利用できると期待される。
概説
疾患予測は、様々な生物学的データを用いた機械学習アプローチによって行われてきた。
代表的なデータの1つは、宿主の免疫システムと相互に作用する、腸の微生物群だ。
多数の微生物が、さまざまな疾患を予測するためのマーカーとして使用されてきた。
本研究では、機械学習アプローチを使用した多重分類によって、多発性硬化症、若年性特発性関節炎、筋肉性脳脊髄炎/慢性疲労症候群、後天性免疫不全症候群、脳卒中、結腸直腸癌という6つの疾患から腸内細菌叢を区別できると仮定した。
最良の予測モデルを確立するために、さまざまな研究から収集された696のサンプルの特徴として、5つの分類レベルで多数の微生物を使用した。
4つのマルチクラス分類器、および変数増加法(前進的選択法)と変数減少法(後退的選択法)を含む2つの特徴選択法に基づいて分類モデルを構築した。
その結果、分類レベルが低いほど、分類のパフォーマンスが向上することがわかった。
最高のパフォーマンスは属レベルにおいて観察された。
4つの分類器のうち、LogitBoostベースの予測モデルが他の分類器と比べて最も優れていた。
また、変数減少法によって得られた属レベルにおける、最適な特徴の部分集合を我々は提案した。
選択された特徴の部分集合は、様々な疾患を同時に区別するためのマーカーとして使用されうると我々は考える。
この研究における発見は、選択された特徴が、複数の疾患の診断に使われる可能性があることを示唆している。
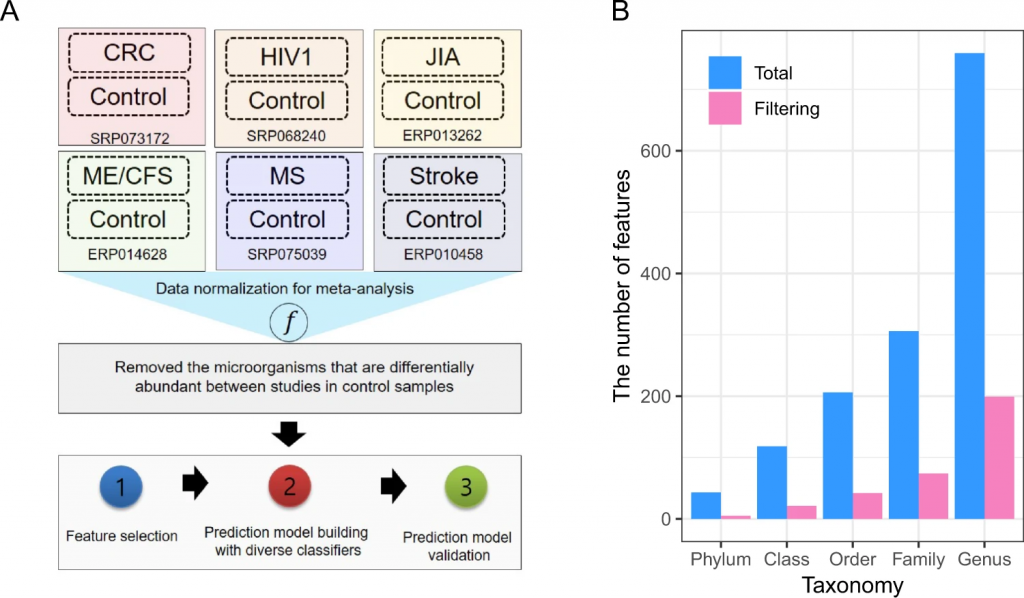
(A)本研究の実験計画全体を表す図。
(B)5つの分類レベルにおける特徴の数。
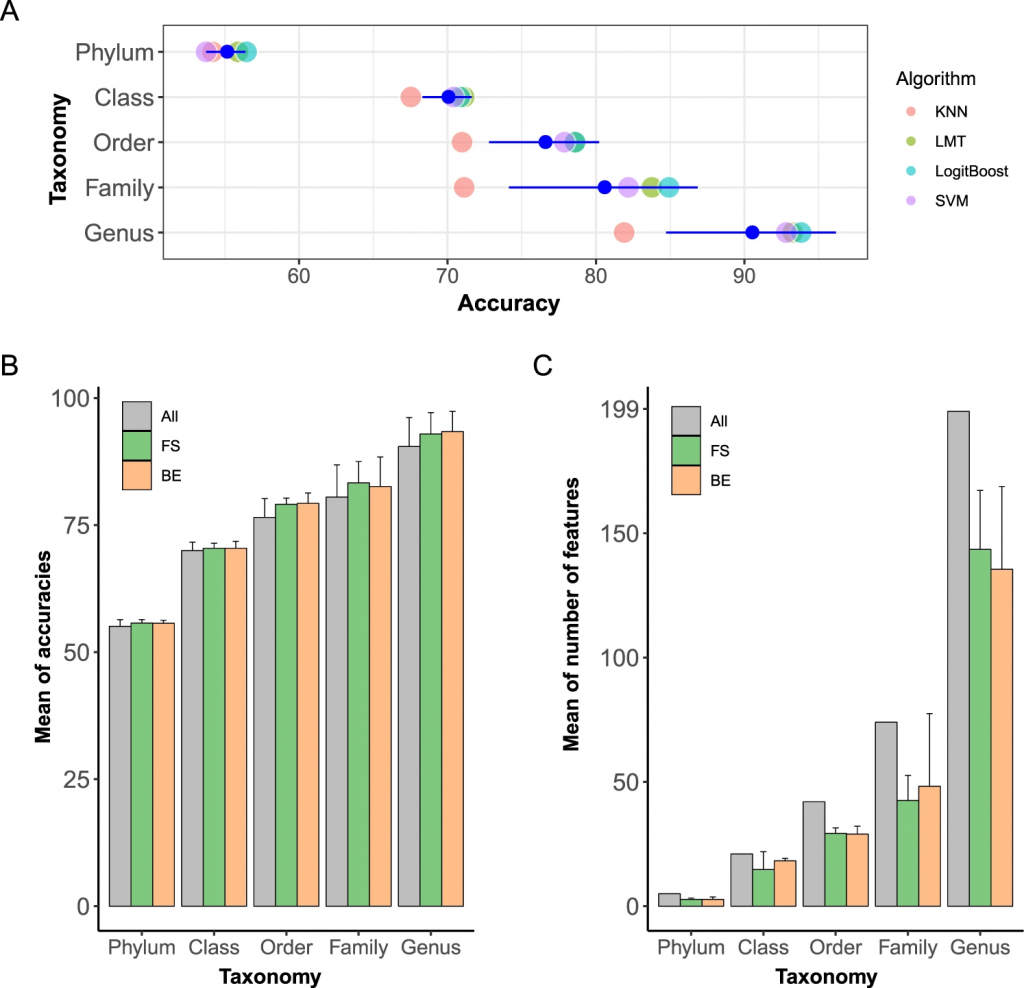
(A)分類レベルごとの各分類器の分類精度とその平均。
(B)分類レベルと特徴選択法による各分類器の精度の平均。
(C)分類レベルごとの各分類器における特徴の数および特徴の選択方法の平均。
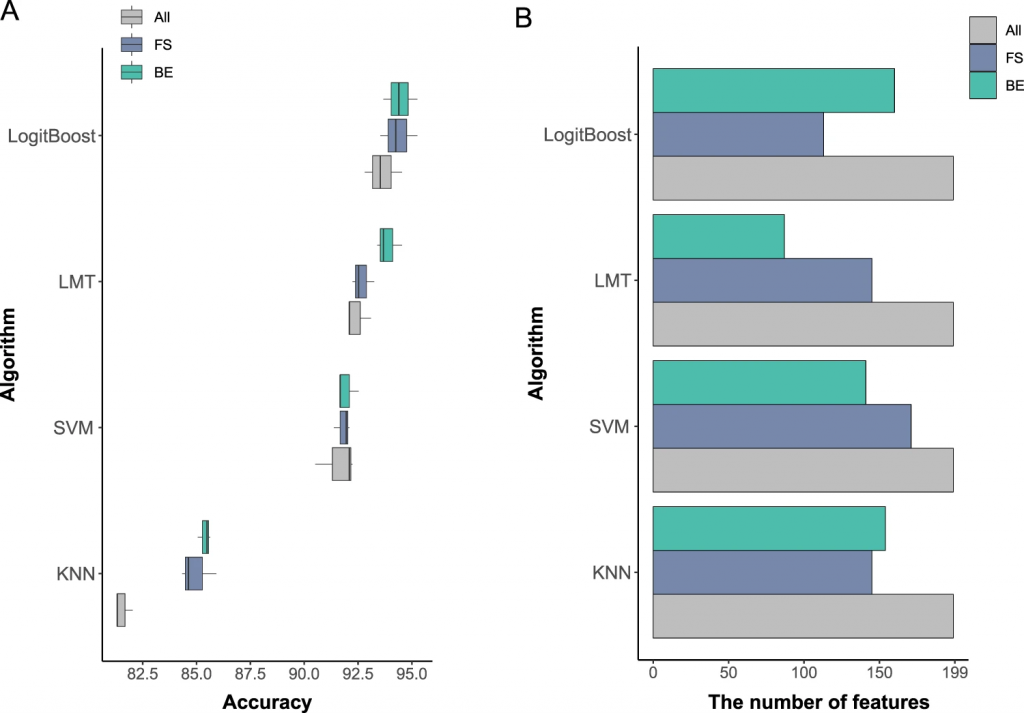
(A)3つの特徴選択戦略を用いた各分類器の精度。
(B)3つの特徴選択戦略を用いた各分類器の特徴の数。
著者
Sohyun Bang (Interdisciplinary Program in Bioinformatics, Seoul National University, Seoul, 151-742, Republic of Korea / C&K genomics, Seoul National University Research Park, Seoul, 151-919, Republic of Korea)
DongAhn Yoo (Interdisciplinary Program in Bioinformatics, Seoul National University, Seoul, 151-742, Republic of Korea)
Soo-Jin Kim (Department of Agricultural Biotechnology and Research Institute of Agriculture and Life Sciences, Seoul National University, Seoul, Republic of Korea)
Soyun Jhang (Interdisciplinary Program in Bioinformatics, Seoul National University, Seoul, 151-742, Republic of Korea / C&K genomics, Seoul National University Research Park, Seoul, 151-919, Republic of Korea)
Seoae Cho (C&K genomics, Seoul National University Research Park, Seoul, 151-919, Republic of Korea)
Heebal Kim (Interdisciplinary Program in Bioinformatics, Seoul National University, Seoul, 151-742, Republic of Korea / C&K genomics, Seoul National University Research Park, Seoul, 151-919, Republic of Korea / Department of Agricultural Biotechnology and Research Institute of Agriculture and Life Sciences, Seoul National University, Seoul, Republic of Korea)
出版情報
Received: 05 October 2018 / Accepted: 12 April 2019 / Published: 15 July 2019
Open Access This article is licensed under a Creative Commons Attribution 4.0 International License, which permits use, sharing, adaptation, distribution and reproduction in any medium or format, as long as you give appropriate credit to the original author(s) and the source, provide a link to the Creative Commons license, and indicate if changes were made. The images or other third party material in this article are included in the article’s Creative Commons license, unless indicated otherwise in a credit line to the material. If material is not included in the article’s Creative Commons license and your intended use is not permitted by statutory regulation or exceeds the permitted use, you will need to obtain permission directly from the copyright holder. To view a copy of this license, visit http://creativecommons.org/licenses/by/4.0/.
Reprints and Permissions
■サポートのお願い
AIDBを便利だと思っていただけた方に、任意の金額でサポートしていただけますと幸いです。